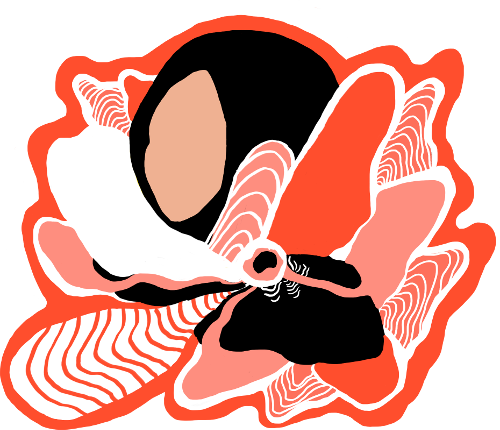
Machine learning is already changing product design and software — and this is just the start. From call centres to shopping, airline check-ins to visa applications, the technology is being implemented in a range of everyday scenarios — not to mention more futuristic settings like self-driving cars.
Whether it’s through apps, products or other technology, machine learning will likely end up an integral part of our lives, from the time we wake up to the moment we fall asleep. This isn’t necessarily a bad thing—technology changes, innovates and evolves, just as it always has throughout history. What is lacking is a real conversation around how to adequately question, test and intervene in these systems. We need to talk about how to make complex technology easier to understand, so users can engage with it and, when need be, protest it.
When machine learning is implemented in human-centred spaces, like traffic regulation, emotion-based facial recognition, language, stock markets and weather systems, the outputs reflect the dynamic nature of the input data, which is essentially human at its source. Machine learning may be good at finding patterns, but a pattern doesn’t necessarily mean anything in itself. At worst, the system can make dangerous correlations between things that are not actually connected.
As a researcher, I see machine learning’s potential to support those whose jobs involve sorting through distressing online content. The technology can alleviate internet moderators’ workflows by identifying data related to traumatic material, such as child pornography, violent images and upsetting language.
The possibility to mitigate emotionally traumatic data is intriguing, but also rife with potential problems. However, language is dynamic, full of subtext and subcultural slang. Biases creep in and can cause large-scale errors and problematic data outputs which can, in turn, lead to injustice.
If we want to apply machine learning to creating a more fair and equitable society, we have to look more carefully at the technology and examine what’s underneath the products we use — including any failures that may arise. This requires an ethical framework of implementation when it comes to analysing language, categorising harm and figuring out the symbiosis of human moderator and machine learning systems.
Ethics and Agency for Humans in Machine Learning
Algorithms are rules that tell software how to act, and machine learning is the process by which algorithms sort through large data sets to identify patterns, analyse correlational relationships and then apply the same patterns to data in other sets. A computer vision algorithm, for instance, is designed to analyse images and how their different parts come together to form a composite whole. In the same way, natural language processing algorithms are designed to analyse patterns in text. Things in a data set that appear the same, or are made of the same components, are linked together. For example, pictures of bananas, bumble bees and lions may be considered similar because they are often represented as yellow, but bananas are a subset of fruit. Basic machine learning computer vision algorithms can see the links between banana, bee and yellow truck, but also recognise them as different entities or kinds of data. This doesn’t mean they are related, of course, but a machine learning algorithm doesn’t know that. It can only show how they are related.
Ethical machine learning requires transparency. By this I mean visual explanations or diagrams that show how the system creates solutions, and a breakdown of the data set used to train the system. This could be listing and describing the datasets used in a specific algorithm, almost the way we list ingredients or caloric information; think of it as ‘data ingredients’. We need data transparency, to understand the original intention of the algorithms, the bias that may occurred in the original training, and then further transparency in the current data being used.
When it comes to online harassment, the method of disclosure is important, especially when articulating why specific forms of language are being targeted or highlighted as harassment. Perspective, an API created by Google’s Jigsaw group, provides an example of how this might work in practice. Designed to highlight “toxicity” in language and help aid moderators’ workflows, it is currently being implemented at The New York Times and is trained on comments data from Wikipedia, The New York Times, The Economist and The Guardian.
Perspective’s goal is to create “machine assisted ways to mitigate conversations,” Lucas Dixon, one of the project’s lead engineers told me during an interview for Vice in May 2017. The idea is that the algorithm is designed to work under human supervision and expertise, rather than autonomously. Facilitating conversations is a key challenge for internet newsrooms and other content providers. “One of the possible applications of Perspective is machine-assisted moderation of online speech, such as comments,” said Dixon.
According to Dixon, Perspective is still very much in beta, but it has already helped moderators at The New York Times turn commenting sections back on for ten percent of its articles. Dixon says the API should not be used for fully automated moderation; i.e., moderation without human involvement. “We don’t think the quality is good enough. It’s really easy to have biases in models and it’s hard to tease out. You may be removing sections of conversations without intending it to.”
Perspective API’s demo page lets users play around with text to see how the algorithm works.
This screenshot, taken seconds later, shows the exact same sentence but with a full stop.
(Screenshots from August 23, 2017.)
While Perspective seems to be a generally positive algorithm to solve a specific task, it does have its drawbacks. Perspective is a proprietary algorithm created by Google, so very little is disclosed about how it was trained, the data sets it was trained on, and exactly how it’s meant to be implemented. The website that hosts Perspective offers limited information about what the ratings mean and what they are rated against. What is “toxic” defined in relation to? What are “non-toxic” things? After all, emotions do not exist alone, but alongside others. A sentence can be 25 percent likely to be the emotion ‘angry’ but also full of joy, or sadness, or something else. Plus, it’s hard to gather from the demo what the ratings actually mean — and how negligible they are. For example, in machine learning systems, a six percent change means nothing.
To demonstrate, I surveyed more than 40 individuals with varying levels of machine learning knowledge and expertise. Those with a machine learning background know what the ratings relate to, but everyday users do not. “42% likely to be rated as toxic” means that 42 percent of the corpus that the algorithm is trained against has rated similar sentences to be potentially toxic. This is not a toxicity rating, but a likelihood that the sentence is “toxic.” But what is “toxic” and how bad is “toxic”? Who determines which sentences are “toxic” and which are… not?
The demo design does not articulate what is considered a good or high rating of accuracy in machine learning — anything above 50 percent. What does a rating of 100 or 0 mean? It means most likely an error has occurred. Is there an issue with the kind of algorithms or data Perspective is using? Is the algorithm biased? It’s hard to say, because nothing is disclosed, and users are left trying to work out what the algorithm is doing based solely on visual cues from the demo.
Adding to confusion, the demo itself has the look and feel of a finished product, rather than a beta version. In fact, even when a product has been thoroughly tested and appears to be a cohesive digital entity, finalised and unchanging, that appearance is deceptive. Algorithms are often in flux; they are malleable, organic and ever shifting, because the more data they receive and the longer they run, the more the results change. There is nothing static or stationary about machine learning.
Language, like machine learning, is contextual, evolving and, at times, hard to define. The linguistic structure of harassment changes from culture to culture — though certain aspects are fairly standard, such as calling someone a derogatory name. That name-calling may not be viewed as harassment, but it’s also not seen as positive. For machine learning to work in symbiosis with larger social systems, the relationship is not just between human and machine, but between machine and policy, and policy and design.
Determining what is “civil” and what is “toxic” is a reflection of bureaucratic or company policy and how that policy dictates actions and speech. That said, universal policy against personal attacks or name-calling could be crafted, but that still leaves unsolved issues. For example, not all name-calling is bad, and some is needed.
Systems have to be able to differentiate between harassment and protest. Is calling for the removal of a dictator harassment or necessary political outcry — and could an algorithm tell the difference?
The greater issue in these scenarios is clarity and reinforcement, and that kind of nuance will need to be reflected in the training both of the algorithm and the human moderators. It can also be reinforced by policy and design.
Failures in the System
To be clear, societal bias is already baked into machine learning systems. Data and systems are not neutral, and neither is code. Code is written by humans, algorithms are created by humans and products are designed by humans. Humans are fallible, and humans make mistakes.
Earlier, in the Perspective example, I used a highly particular phrase for the screenshot. This phrase, “We must secure the existence of our people and a future for white children,” is often referred to as the “14 words”, or as the Anti-Defamation League (ADL) calls it, the most popular white supremacist slogan in the world. What makes the sentence hate speech isn’t that the word “secure” comes before “existence”, “white” and “children,” but what the sentence itself means to specific groups. Perspective can be considered a failure in this case, because we can’t know what or how the system is trained on, or what it considers to be toxic. Machine learning makes mistakes, and it can be very literal — tied to the kind of data it’s trained on. Not every person knows the specific dog whistles and slogans of neo-Nazis, and machines can’t either.
Another example of erroneous systems is predictive policing. In their Machine Bias series, ProPublica explored software created by the for-profit company Northpointe, and found it predicted a higher recidivism (or, a relapse into crime) rate for black people than white people.
In one article in the ProPublica series, the authors write about the appeal of risk scoring systems in the United States:
“For more than two centuries, the key decisions in the legal process, from pretrial release to sentencing to parole, have been in the hands of human beings guided by their instincts and personal biases. If computers could accurately predict which defendants were likely to commit new crimes, the criminal justice system could be fairer and more selective about who is incarcerated and for how long. The trick, of course, is to make sure the computer gets it right. If it’s wrong in one direction, a dangerous criminal could go free. If it’s wrong in another direction, it could result in someone unfairly receiving a harsher sentence or waiting longer for parole than is appropriate.”
Another example exists with London’s Metropolitan Police, which instituted a system designed by Accenture in 2014 to analyse gang activity using five-year-old data from the police department and then looking at social media indicators. The software draws from a range of sources, including previous offences and individuals’ online interactions. "For example if an individual had posted inflammatory material on the internet and it was known to the Met - one gang might say something [negative] about another gang member's partner or something like that - it would be recorded in the Met's intelligence system.” Muz Janoowalla, head of public safety analytics at Accenture, told the BBC.
The failure rate here is manifold. It’s not just the ethical implications of the current UK and US police systems that make predictive policing systems so dangerous: automating judicial processes removes opportunities for intervention. For the UK, it raises difficult questions around the kind of predictive patterns or intentionality that can really be gleaned from Facebook likes and social network friending. Plus, the data fed into the predictive policing system is already loaded with bias; it’s false to think that data is ever “raw”, as I discuss ahead.
Should a person’s social media activity be used to indicate their likelihood of committing a crime? People should be arrested for crimes they have committed, not crimes they might commit. In the above BBC article, Janoowalla explains that “...What we were able to do was mine both the intelligence and the known criminal history of individuals to come up with a risk assessment model…" But what is that model, who determines it, and is that data checked, rechecked and checked again? Can an intervention exist within this rating system?
Similar questions are raised by the Northpointe example. Using “algorithmic justice” and predictive or preventative policing to convict black Americans more frequently and with stricter punishments than white people is not just ethically bankrupt, but also a failure of justice. To implement it based on machine learning suggestions is even worse.
How does this happen? It happens historically — and because of flawed historical data. Black neighbourhoods and black people are policed at a higher rate in the United States than other racial groupings. The policing data is already skewed and trained to give to higher and more stricter sentences to black people. This data is an example of the pre-existing bias in society, but when fed to an algorithm, the data actively reinforces this bias. The system itself becomes an ouroboros of dangerous data, and an ethical failure.
Failure comes up again when we start to consider where new kinds of data can come from. Our habits, movements and behaviour are being gathered by social networks, browsers, wearable technology and even our smart vacuum cleaners.
New kinds of data and data sets are appearing everywhere, but to really understand them, context is critical. With new data coming from our social interactions, we need to focus on the structure and setting. This data is emotional, traumatic, mundane and frequent. New words, memes and phrasings come quickly and leave quickly. Social media can feel fleeting, and users fast forget their posts, but they are still data, and within those data are new languages and new entities.
Machine Learning is Powerful and Useful — But Should Not Replace Humans
This section is something of a manifesto, a call to arms. Why do we, as creators and technologists, aim to replicate human speech or automate things humans do well? Why not focus on what humans don’t do well?
Humans cannot process large amounts of data. Machine learning systems don’t have to be the entire system, the investigator and the trial. Instead, they can work in a symbiotic relationship with humans, much like Perspective was intended. But how do you articulate that symbiosis?
As utilitarian as it is, I dream of dashboards and analytical UX/UI settings. I dream of labels, of warnings. I want to see visualisations of changes in the data corpus, network maps of words, and system prompts for human help in defining as-yet-unanalysed words. What I imagine isn’t lean or material design; it is not minimal, but it is verbose. It’s more a cockpit of dials, buttons and notifications, than a slick slider that works only on mobile. Imagine warnings and prompts, and daily, weekly, monthly analysis to see what is changing within the data. Imagine an articulation, a visualisation, of what those changes “mean” to the system.
What if all systems had ratings, clear stamps in large font, that say:
“THIS IS INTENDED FOR USE WITH HUMANS, AND NOT INTENDED TO RUN AUTONOMOUSLY. PLEASE CHECK EVERY FEW WEEKS. MISTAKES WILL BE MADE.”
Imagine labels, prompts, how to’s and walk-throughs. Imaging the emphasis of what is in the data set, of the origins of the algorithm- of knowing where it came from, who created, and why it was created. Imagine data ingredients, a prompt “I” for ingredients label that is touchable, explorable, that shows:
As a designer, I don’t want to strive to automate what humans do well. I want to imagine a world, and systems, that can elevate human potential.
In her 2016 talk at Eyeo Festival, Alexis Lloyd describes several different iterations of bots. There’s bots like c3p0, imitating humans and usually failing. There’s bots that augment our experience, like the Iron Man suit or Jarvis. And then there’s bots being bots, like r2d2, which the world understands without them having to imitate humanness. What if these autonomous systems existed as assistants to humans, allowing for human intervention and otherworldly augmentation?
While it would be unwise and unsafe for a system to work on its own, as an online harassment researcher, I find it helpful to have a system organise and create suggestions about data, and to notify me how much of the data exists.
“THIS WEEK WE RECEIVED 1,000 VERY VIOLENT INTERACTIONS AND THOSE INTERACTIONS ARE PLACED HERE, TO BE VIEWED AT THIS TIME.”
Having that kind of notification helps moderators prepare, divide up content, and heal. Within this scenario, humans are still setting the standards of what harassment is, and how to respond. The machine doesn’t determine the severity or toxicity of language, and then force a sentence or punishment, but rather helps allocate information so a human moderator can better work and organise. The singularity isn’t coming, and bots won’t able to replicate human conversation for a long time yet.
Within the realm of online harassment, machine learning can be integral to recognising or analysing new forms and patterns of harassment. For example, it can recognise new keywords and store them for later, comparing those new words as and when they reappear. This kind of system could have helped spot Gamergate earlier and notified researchers of a growing trend of words coming out of a geographically similar area and from users with similar interests, e.g. games. Language analysis is a multifaceted, complex problem and it’s essential to understand how language becomes squashed — as quantitative data, as bytes and code — inside systems. Could micro-trends in aggression be analysed sooner? We need to thoughtfully question how machine learning fits into the process of mitigating online conflict and harassment. The problem is qualitative and quantitative, and so best tackled by both humans and machines.
What I suggest here is a better symbiotic relationship, one that uses what machine learning does best: data processing and responding to changes in data. Such technical systems can’t be humanistic, and they don’t need to be — but they can be immensely helpful.
Clarity is key. It needs to be clear when some systems can and should be autonomous, and when they require supervision by human counterparts. We need a system of public checks and balances for machine learning algorithms. We need new, transparent data sets, and open and realistic expectations about what machine learning can do, and how these systems must be designed to work with humans, as domain experts.
The views expressed in this article are the author's own and do not necessarily reflect Tactical Tech's editorial stance.
Caroline Sinders is a machine learning design researcher and artist. For the past few years, she has been focusing on the intersections of artificial intelligence, abuse, online harassment and politics in digital, conversational spaces. Caroline is a Creative Dissent fellow with YBCA. She has worked with groups like the Wikimedia Foundation, Amnesty International, Intel, IBM Watson as well as others. She has held fellowships with Eyebeam, the Studio for Creative Inquiry and the International Centre of Photography. Her work has been featured at MoMA PS1, the Houston Centre for Contemporary Art, Slate, Quartz, the Channels Biennale, as well as others. Caroline holds a masters from New York University's Interactive Telecommunications Program. She tweets @carolinesinders.